Introduction
In this article I will be focusing on where your failure rate data might be coming from, and I will outline some considerations to keep in mind when you perform reliability predictions.
Setting the scene
I’d like you to imagine that you’ve been asked to provide a reliability prediction, but you don’t have a final product, or any data. What would you do? Chances are, you will end up using a prediction standard or a generic database of failure rates, this is totally acceptable given your situation, but do you know how your choice of data limits the utility of your results?
Reliability prediction inputs
It is important to note the two key aspects of any reliability prediction; the model, and the data you feed into that model. When I say model, this could be as simple as summing your failure rates together (assuming they are in series), or a complex RBD (shown below). Regardless of how your model looks, if you are aiming to get a quantitative reliability prediction, there will always be data flowing into your model somehow.
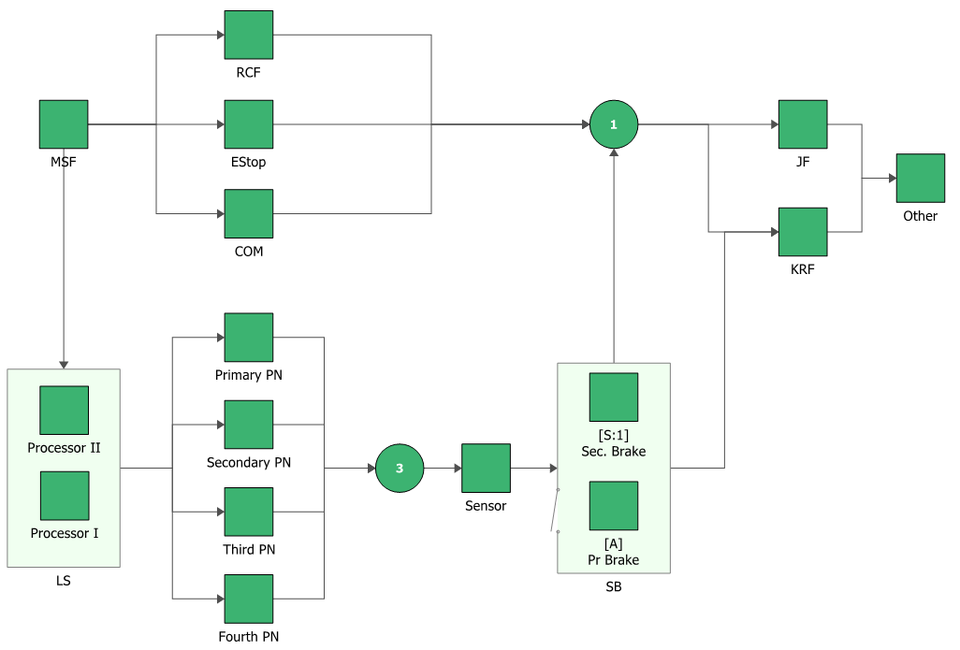
Data sources
We must acknowledge that there are many sources of failure rate data out there, in practice however they may not accessible, relevant or appropriate to your product or analysis. For this reason, I’ll kick things off by listing a hierarchy of data sources for failure rate data. The list is in descending order of preference (best at the top).
Data source | Explanation |
Using In-house field/test data |
|
Using Supplier field/test data |
|
Physics of failure(PoF) methods |
|
Standards based prediction |
|
Generic failure rate database |
|
Assessment criteria
Next we would like some criteria to assess each source against. Depending on the situation some may rank higher than others, and this hierarchy may start to shift. For example, if you have some in-house data (top of our list) but it is outdated and possibly unreliable, then is it any better than a generic database (at the bottom of our list)? To help answer this question I have outlined some points to keep in mind when you sourcing your failure rate data.
Assessment Category | Question to ask |
Data sources | Where has the data come from? |
Type of data and integration with current systems | Is the data relevant and sufficient for our analysis? |
Industry specific data | Is the data relevant to the application/industry of the prediction? |
Maintenance and accuracy | Is the data maintained regularly/at all (the database could be outdated)? |
Security and trust | Do we have confidence in the data we are receiving? |
Putting it to the test
By combining our assessment criteria with our hierarchy of data sources we can be more informed when selecting a data source. As a demonstration, I have populated the table below, let’s imagine I’m working on an electronics product, and the bottom two rows represent MIL-HBK-217F and NPRD-2016 respectively.
| Where has the data come from? | Is the data sufficient? | Is the data relevant? | Is the data maintained and accurate? | Do we have confidence in the data? |
In-house field/test data | 3 | 2 | 3 | 2 | 2 |
Supplier field/test data | 2 | 2 | 2 | 2 | 2 |
Physics of failure(PoF) | 2 | 2 | 1 | 1 | 1 |
Standards based prediction | 1 | 2 | 2 | 1 | 2 |
Generic failure rate database | 1 | 1 | 1 | 2 | 1 |
- 3 = High confidence
- 2 = Medium confidence
- 1 = Low confidence
Reviewing our situation
Ok, so back to the problem, you have no data, your suppliers have no data, and let’s assume you don’t have the tools to perform PoF, then from our table above, it looks like Standards Based Prediction is our best option.
That’s it settled then; we can continue with our reliability prediction! Well, yes, but let’s proceed with caution. The main point here being; what will your prediction tell you? Considering our table screams ‘low confidence’, I wouldn’t recommend you use this result for estimating warranty or maintenance periods. However, besides ticking off a requirement* you could use this result to compare various designs of the product, and flag up any areas that show excessively high contributions to unreliability.
*Reliability should never be a tick box exercise but the reality is that often requirements kick start reliability activities…it’s almost as if this is why reliability has become a requirement.
Summary
My aim from this post was to get you thinking about where your failure data comes from, and to then consider how this impacts how you use your reliability prediction results. I hope this has inspired you to start collecting your own data to help you to climb the hierarchy of data sources so that your reliability predictions can be more insightful in future.
How we can help
Contact us on +44 (0) 333 996 9930 or email: info@wilderisk.co.uk to discuss your specific needs.